Post facilitated by Daniel Maciel, PLD Blog Co-Editor
Whenever and wherever translators meet to talk about the current affairs of their craft, the topic of effective machine translation is inevitable, and that is fine. After all, I cannot think of a more pressing discussion for professional linguists today. However, it is rather odd to see the difficulty in discussing the theme without tying the effectiveness of machine translation to the materialization of true Artificial Intelligence (AI). Actually, achieving the former doesn’t depend on the fruition of the latter. No, fellow translators, it does not. This is a widespread misconception with a lineage that can be traced right back to the long-held general notion of intelligence as a kind of universal and uniform capability. For us translators, such a notion may become an indulgent self-deceit. Please excuse the harsh words, but do bear with me for a moment. I won’t spend much time in lengthy peroration on the nature of AI and Deep Learning; I am also not an expert in the field. I am an educated reader, at best. I think a brief conversation about the relationship between artificial intelligence and machine translation effectiveness will be enough for us to put the elements in the right perspective.
For argument’s sake, let us start by agreeing on intelligence as “the ability to learn or understand or to deal with new or trying situations,” as Merriam-Webster says. In other words, intelligence is the ability to deal with the unknown and then not only learn from it, but also from the process of understanding it. The very way human beings deal with the unknown is a hurdle, as they rely on various subtle sources of input (like emotions or even cognitive biases) to build up their intelligence inventory. Machines do not. Their knowledge is a proxy knowledge in many ways, since they learn from human experience and how humans deal with the world. Even the most recent famous display of “machine intelligence,” when the AlphaGo AI won a series of matches against the best Go player in the world, was, in fact, a demonstration of raw data processing power, not intelligent creativity (or “learning on the go,” so to speak) as we understand it. Now, when we add natural (i.e. human) language and all its nuances and uncertainties to this scenario, it is no surprise that many respectable sources of information on the AI subject consider the idea of having intelligent machines emulate human intelligence in the near future to be an elusive goal.
Still, the crucial question we must ask ourselves is how “intelligent” machines must be to provide effective translations. This is a pesky question, since the answer may imply that it takes little intelligence to translate certain texts. That, of course, is clearly not the case. A translated text is a filigree of complex pieces, each with its own requirements for dealing with novelty, such as context research, terminology accuracy, cultural knowledge, idiomatic adequacy and so on. Likewise, translation is not singular and monolithic. Rather, it is a multifaceted, multi-component phenomenon that may yield an outcome ranging from the most predictable (e.g. a medication leaflet) to the most unpredictable (e.g. poetry). It should go without saying that putting all those pieces together requires a well-developed set of intelligence skills. Yet the more predictable the translation outcome, the more its processes follow certain parameters and the fewer novelties or unexpected variations they involve. This is precisely the environment in which machine translation thrives. I am not saying that predictable, parameterized translation is non-intelligent, “stupid” translation that would not require intelligent translators. What I am arguing is that some types of translation, due to their parameterized nature, provide the raw data volume and the circumscribed environment that machine translation requires to work effectively, much like the game of Go mentioned above or the game of chess, if you prefer. One would hardly say that Lee Sedol or Garry Kasparov are stupid, after all.
That being said, it is easier to understand why translators should not resort to AI’s state of the art as the yardstick with which to measure the impact of machine translation on the translation industry. In human terms, machines that translate do not need to be that “intelligent.” What they must be is robust enough and clever enough to handle vast, sometimes monumental amounts of data in the way we instruct them to do. It means that, depending on the texts to be translated and their position in the range of predictability discussed above, effective machine translation results are not a matter of AI development. They are, actually, a matter of sufficient processing power – achieved through the use of GPUs (Graphics Processing Units) instead of CPUs (Central Processing Units) – applied to texts in very controlled translation environments delimited by well-defined translation constraints at both the linguistic (grammar, lexicon, syntax) and non-linguistic (use, corporate culture, social conveniences) levels. This is how companies or organizations such as the EU, which make extensive and intensive use of machine translation, proceed with such projects. Sure, we have to pay very close attention to everything around AI and the increasingly ubiquitous presence of its incipient forms in our lives. Still, when it comes to machine translation, AI is an umbrella concept, and “true artificial intelligence” is an almost academic discussion. Effective machine translation, on the other hand, is not a concept, but rather a construct consisting of categorical elements derived from specific expectations surrounding clear-cut types of translation. It is not a scientific potentiality; it is a technological reality. It is not an academic discussion; it is already a reality. It may be that you will come to a different conclusion after checking the facts and information yourself. However, as I have said earlier, thinking otherwise without doing that fact-checking is indulging in self-deceit.
Finally, I would like to leave a special word for the dear colleagues who work with transcreation or literary translation. If you think that by “parameterized” and “predictable” I was alluding to the so-called “technical” translation only, think again. There is a significant difference between producing a creative text and recognizing one. The parameters are there, and disciplines akin to translation, like literary criticism and adaptation studies, are looking seriously into how to make machines recognize them. How long it will take, then, for those recognizable parameters to be used in computer tools tailored to your craft? No one knows, of course, but judging from the way things are going, I would risk asserting that many of you may live long enough to see the day.
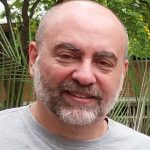
Leave a Reply